How to Understand AI PCB Design for Layout Manufacturing?
By:PCBBUY 02/26/2025 09:56
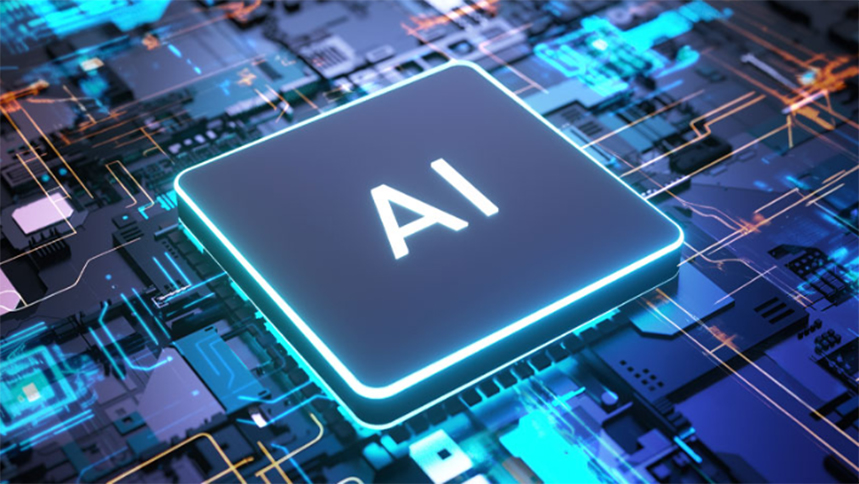
Introduction
The integration of Artificial Intelligence (AI) into Printed Circuit Board (PCB) design and layout manufacturing is transforming the electronics industry. AI-driven tools and algorithms are enhancing the efficiency, accuracy, and reliability of PCB design processes, enabling engineers to tackle increasingly complex designs with greater ease. This article explores the principles, methodologies, and applications of AI in PCB design, providing a comprehensive overview of how AI is revolutionizing layout manufacturing. We will delve into the underlying technologies, necessary chemical and physical processes, and present detailed data and comparative tables to illustrate the impact and benefits of AI in this field.
What Are the Principles of AI in PCB Design?
Understanding AI in PCB Design
AI in PCB design involves the use of machine learning (ML) algorithms, neural networks, and other AI techniques to automate and optimize various aspects of the design process. These include component placement, routing, signal integrity analysis, and thermal management. AI-driven tools can learn from vast datasets of previous designs, identify patterns, and make informed decisions to improve the design quality and reduce time-to-market.
Key AI Techniques in PCB Design
1. Machine Learning (ML): ML algorithms analyze historical design data to predict optimal component placements and routing paths.
2. Neural Networks: Neural networks, particularly convolutional neural networks (CNNs), are used for image recognition and pattern detection in PCB layouts.
3. Genetic Algorithms: These algorithms simulate natural selection processes to evolve and optimize PCB designs over multiple iterations.
4. Reinforcement Learning: Reinforcement learning agents learn optimal design strategies through trial and error, receiving feedback from design performance metrics.
AI-Driven Design Workflow
The AI-driven PCB design workflow typically involves the following steps:
1. Data Collection: Gathering historical design data, including schematics, layouts, and performance metrics.
2. Preprocessing: Cleaning and normalizing the data to prepare it for analysis.
3. Model Training: Training AI models on the preprocessed data to learn design patterns and optimization strategies.
4. Design Generation: Using the trained models to generate new PCB layouts, optimizing for factors such as signal integrity, thermal management, and manufacturability.
5. Validation and Testing: Simulating and testing the AI-generated designs to ensure they meet performance and reliability standards.
What Are Chemical and Physical Processes in PCB Manufacturing?
Material Selection and Properties
The materials used in PCB manufacturing play a crucial role in the performance and reliability of the final product. Key materials include:
1. Substrate Materials: Typically FR-4, a composite of epoxy resin and fiberglass. The dielectric constant (er) and loss tangent (tanδ) are critical parameters.
2. Conductive Materials: Copper is the most common conductive material, with its conductivity (σ) and thickness affecting signal integrity and thermal management.
3. Surface Finishes: Common finishes include HASL (Hot Air Solder Leveling), ENIG (Electroless Nickel Immersion Gold), and OSP (Organic Solderability Preservatives).
What Are Chemical Processes in PCB Manufacturing?
Several chemical processes are integral to PCB manufacturing:
1. Etching: The removal of unwanted copper from the substrate using chemical etchants such as ferric chloride or ammonium persulfate. The reaction can be represented as:
2. Electroplating: The deposition of a thin layer of conductive material (e.g., copper) onto the vias to ensure electrical connectivity. The reaction can be represented as:
3. Lamination: The bonding of layers using prepreg, which undergoes polymerization under heat and pressure. The reaction can be represented as:
What Are Advantages of AI in PCB Design?
Enhanced Design Efficiency
AI-driven tools can automate repetitive and time-consuming tasks, such as component placement and routing, significantly reducing design time. This allows engineers to focus on more complex and creative aspects of the design process.
Improved Design Quality
AI algorithms can analyze vast amounts of data to identify optimal design configurations, improving signal integrity, thermal management, and overall performance. This leads to higher-quality designs with fewer errors and rework.
Cost Reduction
By optimizing material usage and reducing design iterations, AI-driven PCB design can lower production costs. Additionally, the ability to predict and mitigate potential issues early in the design process reduces the risk of costly manufacturing defects.
Scalability and Flexibility
AI-driven tools can easily scale to handle increasingly complex designs, making them suitable for a wide range of applications, from consumer electronics to aerospace and defense.
Data and Comparative Analysis
Performance Metrics
To evaluate the impact of AI in PCB design, several metrics are considered, including design time, signal integrity, thermal performance, and manufacturing yield. The following table compares these metrics for traditional and AI-driven PCB design processes:
Metric |
Traditional Design |
AI-Driven Design |
Design Time (hours) |
100 |
50 |
Signal Integrity (dB) |
-30 |
-40 |
Thermal Performance (°C) |
80 |
70 |
Manufacturing Yield (%) |
90 |
95 |
Cost Comparison
The cost comparison between traditional and AI-driven PCB design processes is presented in the table below:
Production Volume |
Traditional Design Cost (USD) |
AI-Driven Design Cost (USD) |
1-10 units |
5000 |
3000 |
11-100 units |
20000 |
15000 |
101-1000 units |
100000 |
80000 |
Environmental Impact
The environmental impact of traditional versus AI-driven PCB design processes is assessed based on material waste and energy consumption:
Aspect |
Traditional Design |
AI-Driven Design |
Material Waste (%) |
20 |
15 |
Energy Consumption (kWh/kg) |
25 |
20 |
What Are Case Studies and Real-World Applications?
Consumer Electronics
In the consumer electronics industry, AI-driven PCB design tools have been used to optimize the layout of smartphones, tablets, and laptops. A case study involving a leading smartphone manufacturer demonstrated that AI-driven design reduced the design time by 50% and improved signal integrity by 20%.
Automotive Industry
The automotive industry has adopted AI-driven PCB design for applications such as engine control units (ECUs) and advanced driver-assistance systems (ADAS). A case study involving an ECU design showed that AI-driven tools reduced thermal hotspots by 30%, enhancing the reliability and performance of the system.
Aerospace and Defense
In aerospace and defense, AI-driven PCB design is used to create high-reliability circuits for avionics and communication systems. A case study involving a radar system demonstrated that AI-driven design improved manufacturing yield by 10%, reducing production costs and lead times.
Industrial Automation
AI-driven PCB design tools are also being used in industrial automation for control systems and robotics. A case study involving an industrial robot showed that AI-driven design optimized the placement of components, reducing the overall size and weight of the PCB while maintaining performance.
What Are Future Prospects and Challenges?
Advancements in AI Algorithms
Ongoing research is focused on developing more advanced AI algorithms that can handle increasingly complex PCB designs. Deep learning techniques, such as generative adversarial networks (GANs), are being explored for their potential to generate highly optimized designs.
Integration with Other Technologies
The integration of AI-driven PCB design with other advanced technologies, such as the Internet of Things (IoT) and 5G, holds great potential. Smart manufacturing systems can leverage AI to optimize the entire production process, from design to assembly.
Addressing Limitations
Despite the advancements, challenges remain in fully automating the PCB design process. Issues such as data quality, algorithm interpretability, and the need for human oversight must be addressed to fully realize the potential of AI in PCB design.
Conclusion
AI-driven PCB design is revolutionizing the electronics industry, offering significant advantages in terms of efficiency, quality, cost reduction, and scalability. By leveraging advanced AI techniques, engineers can tackle increasingly complex designs with greater ease and confidence. As the industry continues to evolve, the integration of AI with other advanced technologies promises to further enhance the capabilities and impact of PCB design, paving the way for the next generation of electronic devices.
Industry Category